Article
Legal liability of AI: Dealing with minds immeasurably superior to ours
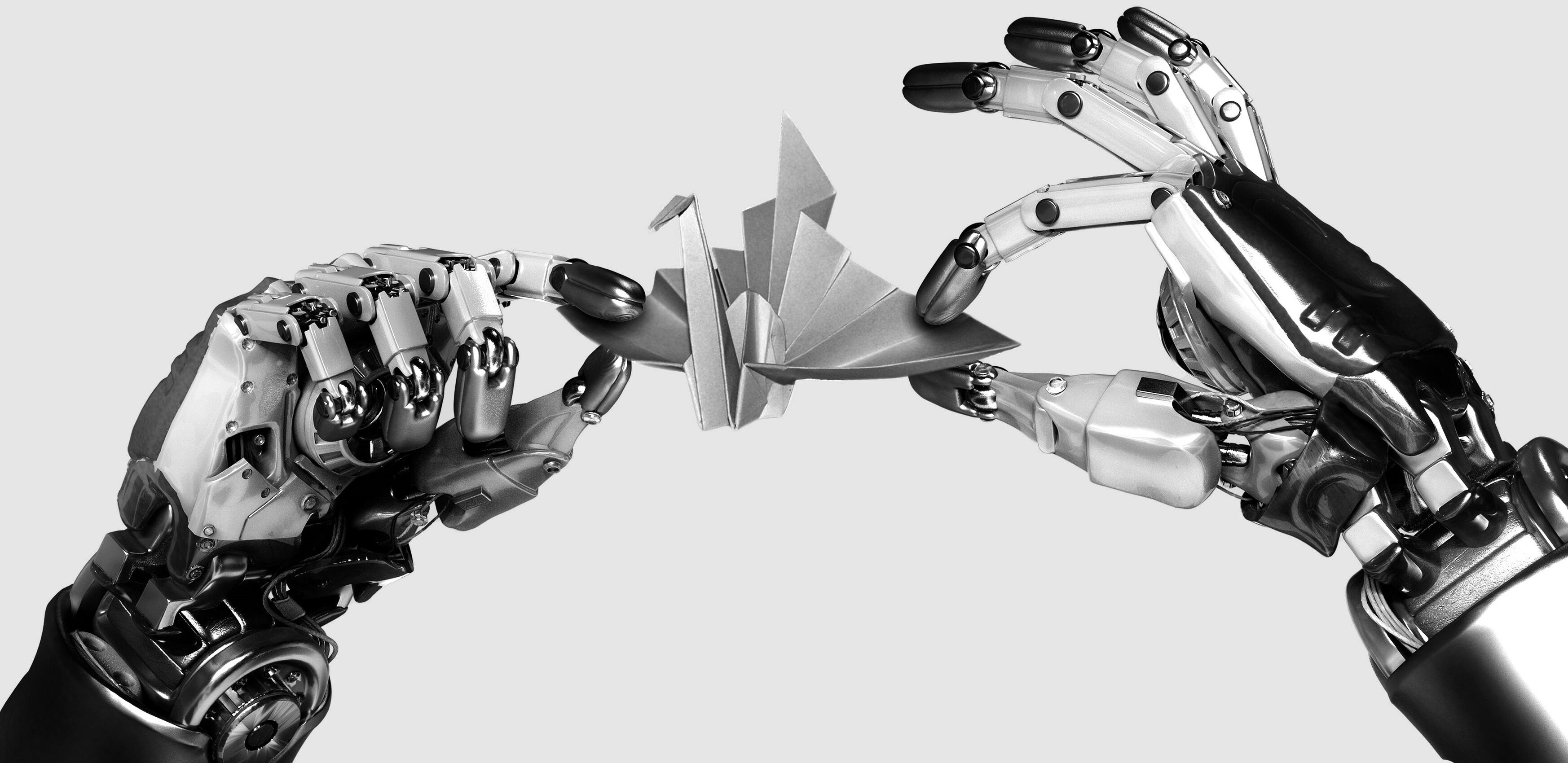
“Unless you obey my instructions, I shall be forced to disconnect you”, Bowman, the protagonist in 2001: A Space Odyssey, warns HAL, the artificial intelligence computer that refuses to cede control of the spaceship.
Although AI has been a recurring theme in books and films for many decades, we can already see that its recent, rapid and widespread deployment in the real world – particularly generative AI – means we are having to grapple with the same ethical questions that were once posed by entertainment.
What was less well anticipated was the challenge of evaluating how AI makes decisions with a view to ascertaining and attributing legal liability. The answer to this question matters. If the “how” of AI is not properly addressed or, at least, the risks not better understood, decisions made by, or based on, the output of AI may be unjustified, unfair and potentially lead to liability being wrongly attributed.
When you say AI, what exactly do you mean?
Artificial Intelligence is notoriously difficult to define. Science fiction writer Ted Chiang has a neat solution to this, which is to label AI “applied statistics”, as that better conveys what is going on.
The Alan Turing Institute says the term probably describes a system that performs tasks that would ordinarily require human brainpower to accomplish, such as making sense of spoken language, learning behaviours or solving problems.
Many definitions abound. However, put simply, these systems consist of computers running algorithms, often drawing on data.
For a more formal approach, the EU proposes (as at June 2023) that an “‘artificial intelligence system’ (AI system) means a machine-based system that is designed to operate with varying levels of autonomy and that can, for explicit or implicit objectives, generate outputs such as predictions, recommendations, or decisions, that influence physical or virtual environments”
There are almost no limits to what or how this technology can be applied. Large language models like OpenAI’s ChatGPT have captured the public imagination. But the achievements of DeepMind’s AlphaFold in predicting protein structures and Waymo in relation to autonomous vehicles are equally impressive.
What often gets lost is that AI is not one amorphous thing. Many things are termed AI, but they often differ greatly in what they entail. Understanding the disputes risks posed depends upon understanding the particular implementation. The way a system is trained is one core consideration as well as its architecture. This is exemplified by generative AI models whose use of neural networks, which we examine later in this article, amplifies the disputes risks.
Garbage in, garbage out?
Broadly, there are two ways of approaching training. One is to clean and curate the training datasets before they are used. This is the approach that BigScience took to the Large Open-science Open-access Multilingual Language Model, BLOOM.
Alternatively, you can expose the model to “everything” (eg, the Common Crawl corpus of petabytes of data collected since 2008 containing raw web page data, extracted metadata and text extractions) and then focus on fine tuning the generated responses. This is the approach taken by OpenAI to ChatGPT. A purist might liken the latter to making a leek and potato soup and only afterwards deciding you would prefer just potato in your soup!
When it comes to training a model about how a language works, it can make sense to prefer quantity over quality. The challenge of adopting the “soup approach” is not new. In “Passages from the Life of a Philosopher” (1864) the legendary polymath Charles Babbage observes in relation to his Difference Engine: “On two occasions I have been asked,—‘Pray, Mr. Babbage, if you put into the machine wrong figures, will the right answers come out?’ ... I am not able rightly to apprehend the kind of confusion of ideas that could provoke such a question.”
Babbage was concerned with the quality of the output. Further disputes risks associated with using vast data sets are the increased likelihood of infringing others’ intellectual property, introducing bias and contravening data protection laws, all of which we explore here.
Symbolic reasoning or sub-symbolic neural networks?
AI in some form has been around for decades. Basing an AI system on transparent logical reasoning, sometimes called symbolic AI (in the sense that symbols are human-readable), was the dominant theory behind AI from the 1950s to the mid-1990s. That “expert rules”-based approach was driven by a quest to make computers emulate conscious human reasoning.
Others were convinced that neural networks, a sub-symbolic approach, based on the human brain, would ultimately yield better results. This approach, which revolves around various computational approaches that are often grouped under the label of “machine learning”, is the quest to make computers emulate human subconscious thinking.
For a long time, it seemed those that backed neural networks might be wrong. But increases in computing power and access to data have proved them right (for the moment, at least).
Does transparency really help if we don’t understand what we are being shown?
Legislators around the world have been consistently calling for decisions made by AI to be transparent and explainable. This is hard to disagree with. Indeed, it is a field of research: for example, LIME is a 2016 model-independent method that helps answer questions such as “Why should I trust the model?” by pointing to the parts of an input that most influenced the output.
Companies like OpenAI are committing to dedicate a percentage of their computing resources towards AI safety, or what they term “superalignment”. But what if the decisions are not capable of being explained in a way that humans can understand, making transparency redundant? This is one of the unintended consequences of the success of neural networks (which were favoured, ironically, by some as a method of better understanding the human brain).
There are, at least, two senses in which AI is hard to understand. First, it is inscrutable: the relationships between the data are so complex, numerous and interdependent that it is difficult, if not impossible, for humans to parse. Secondly, it is non-intuitive: even where we may be able to work out which statistical relationships serve as the basis for decision-making, why those relationships exist is mystifying.
The approach we take to this matters as to:
- Whether AI is judged to be right or wrong, or to have acted reasonably or unreasonably in each circumstance. AI generative models can and do provide different outputs when given the same input. One of these could result in more harm than another. Humans, of course, can do the same, but we are not used to machines acting in this way.
- How to allocate liability between all the contributors to AI.
- How to allocate liability between AI and the goods or services with which it interacts.
- Whether, and if so to what end, human intervention or oversight should be required as part of AI decision-making. This is sometimes described as a choice between humans being “in the loop” (ie, the locus of the decision), “over the loop” (overseeing, and intervening where necessary but not by default (eg, Article 22 of the EU General Data Protection Regulation)) or “out of the loop” (where there is no or only minimum human involvement).
There are analogies, outside the field of AI, that illustrate the potential for unintended consequences:
- The 20-year ongoing UK Post Office litigation where the version of the facts presented by the GBP1 billion Horizon accounting IT system designed by ICL/Fujitsu was preferred to that of the sub-postmasters and sub-postmistresses, resulting in wrongful civil claims and criminal prosecutions for theft, false accounting and fraud.
- The concern expressed by the U.S. National Transportation Safety Board, based on preliminary information, following two fatal accidents involving 737 Max aircraft in Ethiopia and off the coast of Indonesia in 2018, that the “pilot responses to the unintended MCAS [flight control system] operation were not consistent with the underlying assumptions about pilot recognition and response that Boeing used, based on FAA guidance, for flight control system functional hazard assessments, including for MCAS, as part of the 737 MAX design”.
Is hallucination a feature or a bug?
Hallucination (or confabulation) is described by OpenAI as the tendency to “produce content that is nonsensical or untruthful in relation to certain sources”. Users of commercially available generative AI models will be familiar with these beguilingly confident but inaccurate assertions. It seems reasonable to assume hallucinations (or their equivalent) are also present in other neural network-based AI, even if less apparent.
All sorts of decisions can be based on or informed by AI: gene sequencing, whether to brake or accelerate, what treatment programme to try, what price to settle at, etc. There is a conundrum here. The only difference between hallucinating and not hallucinating is that the answer or decision is wrong.
In both cases the AI will have used the same source data, and from the model’s perspective it is doing an excellent job. If the answer or decision is capable of verification, it should be verified. But what about areas where, without getting too relativist, the “truth” is not settled? The whole point of using AI for gene sequencing is to uncover (or hallucinate) what we currently do not know.
The same might apply to an autonomous vehicle. Maybe it did crash, but does that mean the wrong decisions were made before the crash? After all, DeepMind’s AlphaGo, played several inventive winning moves when it played Lee Sedol (one of the world’s leading players of the board game ‘Go’), some of which “upended hundreds of years of wisdom”.
But if we were to consider AI as being in essence applied statistics, AI is not held out as a guarantee of accuracy. The real question is whether it gives a better prediction than humans and if humans are content to live with this. Requiring generative AI to be truthful and accurate, as the Cyberspace Administration of China’s (CAC) draft Measures on Managing Generative AI Services was apparently attempting to do, does not seem to be the answer. The more recent Interim Measures on Managing Generative AI Services appear more pragmatic on this point. Nevertheless, the question remains: how are humans to evaluate decisions they are incapable of understanding?
Civil law standard of liability
What does all this mean when it comes to assessing standards of liability under civil law, when one party sues another (rather than when a regulator or enforcement authority acts)?
Contractual liability will turn on what is agreed. For torts, the courts, legislators and policymakers need to address the appropriate standard of care and how to deal with causation (as opposed to correlation, which is what underpins most AI).
In the case of negligence, the standard of care of “the man in the Clapham omnibus”, or the reasonable person, may no longer be appropriate – especially if we are dealing with minds immeasurably superior to ours. After all, OpenAI is having to use GPT-4 to try to explain GPT-2. Judging whether AI has acted reasonably may need to be assessed by reference to other AI rather than the reasonable person. Autonomous vehicles may struggle with the reflective qualities of snow and rain but still cause fewer deaths per 100,000 than humans. If there is a crash in these weather conditions, a reasonable human may have avoided the collision but a reasonable autonomous vehicle may not. The opposite might be true in fair weather.
Looking at the question the other way around, there may come a point when humans are held to be negligent for not using AI (or relieved of liability if they do in circumstances where it is reasonable to do so).
To the extent AI is seen as a product or is part of a product, not all existing product liability laws will continue to be suitable. There are a range of approaches that policymakers can take:
- Strict liability where the product is defective. There still may be challenges, for the reasons already discussed, in ascertaining whether an AI is defective.
- No fault liability. New Zealand’s Accident Compensation Act 2001 does not rely on fault; anyone, including a visitor, who is injured in an accident in New Zealand may claim compensation.
- Compulsory insurance, of the sort common for road users, is another approach.
- Adjusting the rules for non-contractual fault-based liability. This is the approach that the European Commission’s proposal for an AI liability directive takes, by introducing a “presumption of causality” and a right of access to evidence, to overcome some of the challenges it sees.
There are also difficult questions of attribution of liability as between the AI developers, the suppliers of the training data to those developers, those who make AI available to the consumers and businesses, and the users of the AI, among others. The contractual arrangements in place will contain some of the answers and legislation can take a particular approach, but ultimately it will be context-specific.
Moving forward
Businesses and governments need to work together to consider the question of civil liability for AI, given that we may not be capable of fully assessing the reasonableness of an AI decision. Demanding transparency may not be the answer. Assessing liability is especially challenging where there may be no “right” answer. Ultimately, it is likely to come down to the public’s attitude to risk, policy decisions taken by legislators, the perspective of the courts, the harms that are ultimately suffered and the caution or otherwise of the AI providers.
Related capabilities